Effective language communication has become more crucial in today’s hyper-connected world, characterized by the widespread use of the internet, mobile devices, and social media. The convergence of Artificial Intelligence (AI) and language translation technology brings us closer to realizing this vision. Meta, the company behind SeamlessM4T, has introduced a groundbreaking multimodal model that promises to transform the landscape of speech translation and transcription. This article delves into the details of SeamlessM4T, shedding light on its capabilities, architectural innovations, and potential to bridge linguistic divides.
A Universal Language Translator: The Challenge
While the concept of a universal language translator has intrigued science fiction enthusiasts for decades, the complexities of language diversity have limited the reality. Existing speech-to-speech and speech-to-text systems only cover a fraction of the world’s languages, posing a challenge to effective communication across linguistic boundaries. SeamlessM4T, however, marks a significant advancement in this arena by addressing the limitations of language coverage and fragmented subsystems.
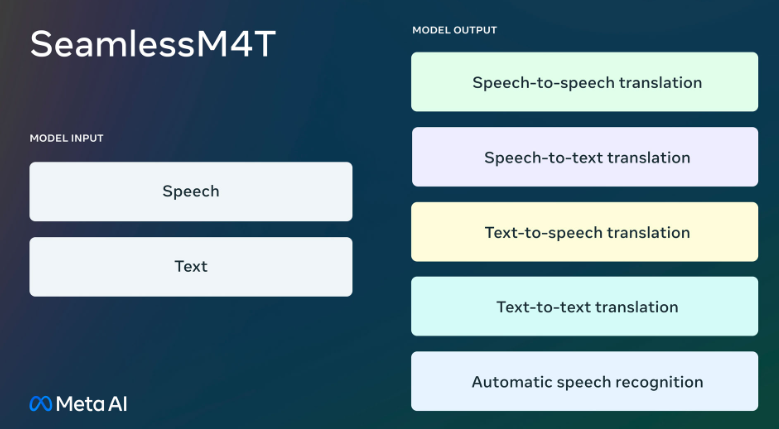
SeamlessM4T: A Multimodal Marvel
The model can translate and transcribe speech and text across various languages seamlessly. SeamlessM4T boasts a comprehensive set of features:
- Automatic speech recognition for nearly 100 languages.
- Speech-to-text translation for almost 100 input and output languages.
- Speech-to-speech translation, supporting approximately 100 input languages and 35 (+ English) output languages.
- Text-to-text translation for nearly 100 languages.
- Text-to-speech translation, spanning around 100 input languages and 35 (+ English) output languages.
Meta’s commitment to open science is evident in its decision to release SeamlessM4T under the CC BY-NC 4.0 license, empowering researchers and developers to build upon this innovative work. Furthermore, the metadata of the expansive SeamlessAlign dataset, comprising 270,000 hours of aligned speech and text, will also be available. The community will engage in their mining efforts using SONAR, a suite of speech and text sentence encoders, and stopes, a multimodal data processing and parallel data mining library supported by Meta’s next-gen sequence modeling library, fairseq2.
The Architecture of SeamlessM4T: Unified Multimodal Approach
Developing a unified multilingual model that addresses various translation tasks requires an advanced architecture. SeamlessM4T leverages the multitask UnitY model architecture, capable of directly generating translated text and speech. The architecture accommodates multiple components:
- Text and speech encoders recognize speech input in nearly 100 languages.
- Text decoder transferring meaning to nearly 100 languages for text, followed by text-to-unit model for 36 speech languages.
- Self-supervised encoder, speech-to-text, and text-to-text translation components, pre-trained for model quality enhancement and training stability.
- The text-to-unit model for discrete acoustic unit generation was later converted into audio waveforms using a multilingual HiFi-GAN unit vocoder.
Processing Speech and Text
SeamlessM4T employs a self-supervised speech encoder, w2v-BERT 2.0, which analyzes multilingual speech to extract structure and meaning. This encoder breaks down audio signals into smaller segments and builds internal representations. Similarly, a text encoder based on the NLLB model has been trained to understand text in nearly 100 languages.
Decoding and Data Scaling
The model’s text decoder can handle encoded speech and text representations, enabling tasks within the same language and multilingual translation. Acoustic units represent speech on the target side, with the text-to-unit component generating discrete speech units based on text output. Data scaling is addressed through pioneering text-to-text mining work, resulting in SONAR, an efficient multilingual and multimodal text embedding space.
Responsible AI with SeamlessM4T
The importance of accuracy, safety, and inclusivity in translation systems is extremely important. Meta adopts a responsible AI framework guided by five pillars. Examining research on toxicity and bias helps identify and address potential pitfalls. Toxicity in both input and output is monitored, with precautions in place to filter and manage toxic content. The model’s performance against background noise and speaker variations demonstrates its robustness.
The Path Forward: Enabling Global Communication
SeamlessM4T’s launch marks a significant milestone in the pursuit of universal communication. Meta’s commitment to open science and innovation promises to reshape how people communicate across languages. As the technology matures, there’s potential for broader applications that transcend linguistic barriers.